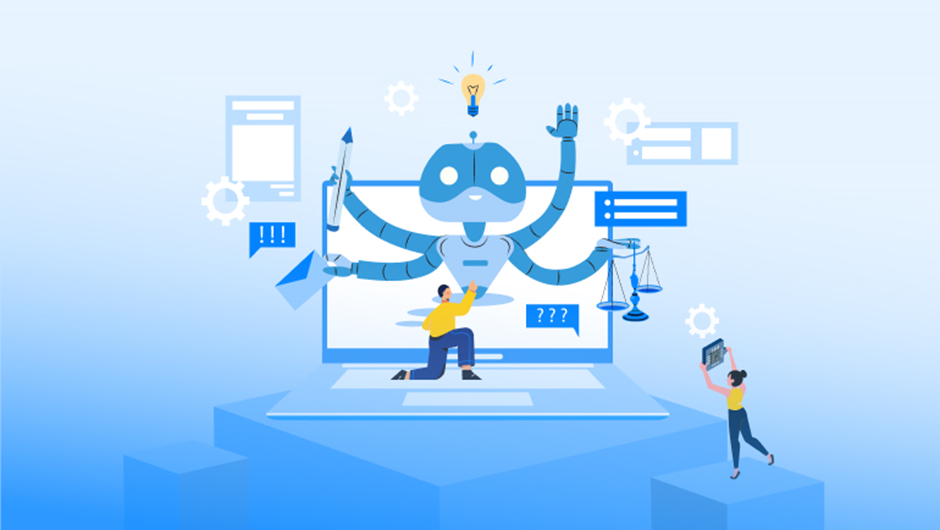
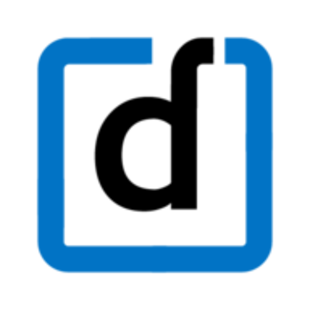
Integrating Artificial Intelligence (AI) in Human Resources (HR) presents ethical and legal challenges, including explainability, bias, and privacy concerns. Responsible AI application necessitates adherence to principles of accountability, explainability, inclusivity, transparency, bias-free, and responsible use. Darwinbox's AI in HR illustrates the importance of ethical and legal compliance. It emphasizes the need for AI systems to be transparent, fair, and unbiased to ensure effective, equitable HR processes.
AI is no longer restricted to science fiction movies or niche experimental projects. It is here for use in our daily lives. And it is here to stay.
There is no doubt that the ‘proof of concept,’ so to speak, has been achieved. The market opportunity is vast, and it is time to examine AI's ethical and legal aspects.
Organizations have already adopted AI across functions and are investing time, money, and resources to integrate AI into their operations. However, it’s essential to pause and reflect on the different aspects of AI and how it can influence how we work – including leaving a negative impact in some instances.
The Need for Responsible AI in HR
In the HR realm, organizations and leaders must do this as the HR function touches every employee and relates to human lives.
Unlike other technical fields that work based on hard facts and data, the world of HR is unique in that it requires decisions based on intangible nuances. If AI algorithms aren’t trained to do this, organizations could wind up with poor and damaged work culture and broken processes.
Here are some problems that organizations might face.
- In HRMS systems, explainability challenges revolve around the opacity of AI decision-making processes. When AI automates recruitment, performance evaluations, or promotions, it's crucial yet difficult to understand how it reaches its conclusions. This lack of clarity can lead to trust issues among employees and management, complicating compliance with legal standards requiring transparency and fairness. Addressing these challenges necessitates developing AI systems that make accurate decisions and provide understandable explanations for their outputs, ensuring ethical and fair HR practices.
- Poorly trained algorithms and AI programs may perpetuate biases and inequalities in the workplace based on flawed data and incorrect stereotypes. A clear example of this is the negative impact of AI on the hiring processes. In 2018, Amazon got into trouble because of an AI-powered experimental hiring tool that discriminated against women. The tool was designed to review job applicant resumes and select top candidates. Still, it consistently downgraded resumes that included the word "women's," as it had learned to penalize resumes that included terms more commonly found on women's resumes. Amazon abandoned the tool after discovering the bias.
- The surge in generative AI and privacy legislation globally demands careful navigation of data protection laws for AI data usage. In the U.S., 13 states have enacted comprehensive data protection laws in under three years, paralleling stricter privacy laws worldwide. These laws often specifically address AI applications, highlighting the complexity of using personal data in AI tools, especially within HR. Employers face challenges disclosing personal data to AI, ensuring compliance with data protection during AI's data handling, and managing data rights requests. This evolving legal landscape necessitates stringent measures to safeguard personal data within AI applications.
As we advance, AI will have a deeper and broader impact on every aspect of the HR function. So, it is time to set the house in order before AI-powered HR becomes all-pervasive and it becomes impossible to put the genie back into the bottle. If not done correctly, and if not done at the right time before it is too late, it could lead to serious, irreversible complications.
Six Key Principles that Define the Ethical Landscape of AI
An excellent way to ensure that things do not go out of hand as AI works for HR function is to be mindful of the fact that while AI-powered tools lift a lot of weight off our shoulders by completing basic tasks, humans are still responsible for how the machine works.
AI essentially works based on human direction, and setting basics in order can help ensure that technology remains responsible.
Irrespective of the industry and function for which AI is used, six fundamental principles must be examined to ensure that AI is as ethical and legally compliant as possible.
Accountability:
- Traceability of Decisions: All HR systems and processes must be designed to ensure that all AI-based decisions are recorded and documented. This traceability is essential for understanding why certain automated decisions were made, such as why a candidate was shortlisted, or an employee was recommended for a promotion.
- Oversight and Responsibility: Even with advanced AI tools, final decision-making in sensitive areas like hiring or employee evaluations must involve human HR professionals. This ensures that a person or group is always accountable for the final decisions.
- Regular Auditing and Improvement: Continuous monitoring and auditing of AI systems is crucial to ensure they function as intended and do not develop or perpetuate biases over time. This involves regularly assessing the AI's performance and adjusting to maintain fairness and accuracy.
- Legal and Ethical Compliance: Adhering to legal standards and ethical guidelines is a fundamental part of accountability in HR technology. AI systems must comply with employment laws and ethical principles, ensuring they do not discriminate and uphold fairness in all HR processes.
- Communication and Feedback Loop: Maintaining open channels for feedback from those affected by AI decisions is essential for accountability. This feedback can help identify areas for improvement and ensure that the AI systems continue to serve the needs and rights of individuals fairly and effectively.
Explainability:
- Clarity in Decision-Making: Explainability refers to the ability of AI systems to articulate the reasoning behind their decisions. Users and stakeholders must understand how the system is trained and the basis on which AI systems make decisions.
- Enhancing Transparency and Trust: Explainability fosters transparency, building trust in AI systems. For example, employees can grasp how an AI evaluates their performance.
- Compliance with Regulations and Ethical Standards: With the growing focus on AI governance, explainability aids regulatory compliance. Many industries are now subject to regulations that demand transparency in automated decision-making.
- Improving AI Systems through Feedback: When an AI system's workings are transparent, users' feedback becomes more informed and valuable. User feedback based on understanding AI recommendations can significantly refine algorithmic performance.
- Addressing Bias and Ensuring Fairness: Explainability is crucial in identifying and mitigating biases in AI systems. Understanding how an AI system arrives at its conclusions is the first step in ensuring these systems are fair and unbiased.
Inclusivity:
- Diverse Data and Perspectives: AI systems should be trained on diverse datasets to reflect the variety of users they serve. This is crucial in industries like HR technology, where recruitment tools must avoid biases against any group.
- Accessible Design: AI tools should be designed to be accessible to users with different abilities and backgrounds. In sectors like education technology or customer service, AI interfaces and interactions should be adaptable to suit various users, including those with disabilities.
- Cultural and Ethical Sensitivity: AI should be developed with an understanding of cultural differences and ethical considerations. This is especially important in HR at global companies where AI needs to navigate diverse cultural norms and values.
- Preventing Discrimination: Inclusivity in AI aims to prevent discriminatory outcomes. Whether in legal tech, finance, or HR, AI systems should be rigorously tested to ensure they do not inadvertently discriminate against any user group.
Transparency:
- Openness About AI Functioning: In HR, transparency about how AI systems make recruitment decisions or performance evaluations is crucial. Understanding AI's role in decision-making is essential for user trust.
- Disclosure of Data Usage: It is important to disclose what employee data is used, where, and how it is used because the HR function houses sensitive personal information that needs to be protected. Transparency in how employee data is handled and consent is obtained is fundamental. This is similarly critical in consumer-facing industries like social media, where AI uses personal data.
- Regulatory Compliance: Transparency in regulatory compliance is essential in HR, especially regarding labor laws and data protection regulations.
Bias-Free:
- Mitigating Bias: In HR, it's essential to configure AI algorithms to avoid discrimination. This can involve careful analysis and adjustment of AI algorithms to prevent discrimination based on gender, race, age, or other factors.
- Diverse Training Data: Using diverse and representative datasets is vital to developing bias-free AI. In HR, this means training AI on data reflecting varied demographics to ensure fairness in hiring or employee assessments.
- Continuous Monitoring: Bias in AI is not always apparent at the outset and can evolve. Regularly reviewing AI systems helps maintain fairness over time, a practice critical in HR tech.
- Regulatory Compliance and Ethics: Adherence to laws and ethical guidelines is critical in developing unbiased AI. In HR, this means compliance with employment and anti-discrimination laws.
Responsible:
- Beneficial Use, Avoiding Harm: Responsible AI means enhancing employee experiences and improving HR processes without causing harm. Across industries, the use of AI should aim for positive, ethical contributions. Responsible AI in HR involves avoiding applications that might lead to unfair discrimination or violate privacy.
- Privacy and Data Security: Maintaining the privacy and security of data in AI applications is essential in HR and is a responsibility that organizations shouldn’t take lightly.
- Societal Impact and Sustainability: Responsible AI use in HR considers the broader societal and environmental impacts. Across industries, aligning AI with sustainability goals and reducing ecological footprints is essential.
- Continuous Learning and Adaptation: Staying informed about the latest AI developments and adapting strategies to be more environmentally sustainable and efficient is part of responsible AI use in HR. Continuous learning and adaptation are vital in fields like AI development and application.
Ethical AI at Darwinbox
Darwinbox has embraced AI in HR functions across the employee lifecycle. Since day 1, we at Darwinbox have ensured that we keep in mind all the six principles that govern the ethical use of AI.
This steadfast focus on being ethically and legally responsible translates into how we build products. For example, Darwinbox has implemented a Retrieval-Augmented Generation (RAG) technique for the helpdesk solution on the Darwinbox HCM platform.
Developing such a system might traditionally involve fine-tuning a large language model (LLM) with proprietary information and FAQs. However, this raises serious security, resources, and efficiency concerns. Darwinbox decided to use the RAG-based system because of the following reasons:
- Enhanced Security: By not feeding proprietary information into the model, there is a reduced risk of sensitive data exposure, even if model artifacts are compromised.
- Up-to-date Information: The RAG system can dynamically pull the latest information from a vector database, eliminating the need for frequent fine-tuning to update the model with new data.
- Reduced Risk of Hallucination: Since responses are generated based on retrieved information, the model is less likely to produce factually incorrect or 'hallucinated' content.
- Lower Computational Resources: Avoiding continuous model retraining reduces the demand for intensive GPU resources, which is cost-effective and energy-efficient.
- Lower Carbon Footprint: Reduced reliance on heavy computational resources translates into a smaller environmental impact, aligning with sustainable and responsible AI usage.
- Flexibility and Scalability: The RAG approach is adaptable and can quickly scale with the growing data and changing platform needs without extensive reprogramming or retraining.
Another example is the use of AI models for recruitment.
As companies become more global, with employees working worldwide, the need for advanced and inclusive Natural Language Processing (NLP) tools is increasingly evident. For this, Custom Named Entity Recognition (NER) models cater to a diverse array of international resumes. The core challenge stems from the model's original training, based on resumes and job descriptions from a specific region, leading to inherent biases when dealing with international data. There would be significant variations in the structure and content of resumes across different geographical areas.
The NER model was re-engineered to recognize and correctly interpret the nuances of resumes from various global regions to address these disparities and bring inclusivity. This included accommodating different educational systems, grading scales, and professional experience representations. The model was also trained to respect privacy norms and cultural differences in personal information disclosure.
As a result, the recruitment model now has:
- Diverse Educational Background Interpretation: Adapting to various global standards depicting education, degrees, and institutions.
- Inclusive Professional Experience Recognition: Accurately parsing job roles and achievements from different resume formats.
- Cultural Sensitivity in Personal Data: Respecting the varying norms of personal information disclosure in resumes.
- Adaptive Format Understanding: Recognizing and interpreting a range of resume formats and design styles.
By adapting the NER model to these diverse global standards, the system became more efficient and accurate and moved towards a more inclusive and bias-free AI solution. This advancement is crucial in today's international job market, ensuring that AI tools contribute positively to global employment practices, transcending regional biases and embracing a global perspective.
By embracing these principles, we're not just harnessing the power of AI but also steering it towards a future where technology serves humanity in its richest diversity and complexity.
Darwinbox’s AI-powered HCM ensures that AI is used ethically and responsibly for every function. To learn more about how Darwinbox uses AI and how our AI-powered HCM can transform your HR operations, schedule a demo and speak to our specialists!
Speak Your Mind